预训练模型
预训练模型 wav2vec2.0、datavec
==Schneider, Steffen, et al. “wav2vec: Unsupervised pre-training for speech recognition.” arXiv preprint arXiv:1904.05862 (2019).==Facebook
思路
- 提出一种用无监督预训练来改善有监督语音识别的方法;训练一个无监督预训练模型,用来给下游的语音识别任务作为输入;
- 该无监督预训练模型叫做wav2vec;该模型输入是原始音频,输出是embedding,这个embedding是用大量无标签音频训练得到的,能表示一定的输入音频信息,模型结构用的CNN;loss function是contrastive loss,从negative中鉴别出哪个是真实的 future audio sample;
方法
输入原始音频x通过encoder网络,得到具有上下文表示的输出z;
The encoder layers have kernel sizes (10; 8; 4; 4; 4) and strides (5; 4; 2; 2; 2).
causal convolution with 512 channels, a group normalization layer and a ReLU nonlinearity.
输入30ms窗长,10ms窗移,16kHz音频,输出低频特征;
然后经过一个context network $g:Z -> C$ ,把encoder的输出z,多个z concat起来经过g得到c:$\large c_i=g(z_i…z_{i-v})$,其中v是感受野,是一个超参;
The context network has nine layers with kernel size three and stride one ,感受野210ms;
causal convolution with 512 channels, a group normalization layer and a ReLU nonlinearity.
group norm:我们发现选择一种对输入的缩放和偏移量无关的归一化方案是很重要的,该归一化方法对于跨数据集泛化性更好;
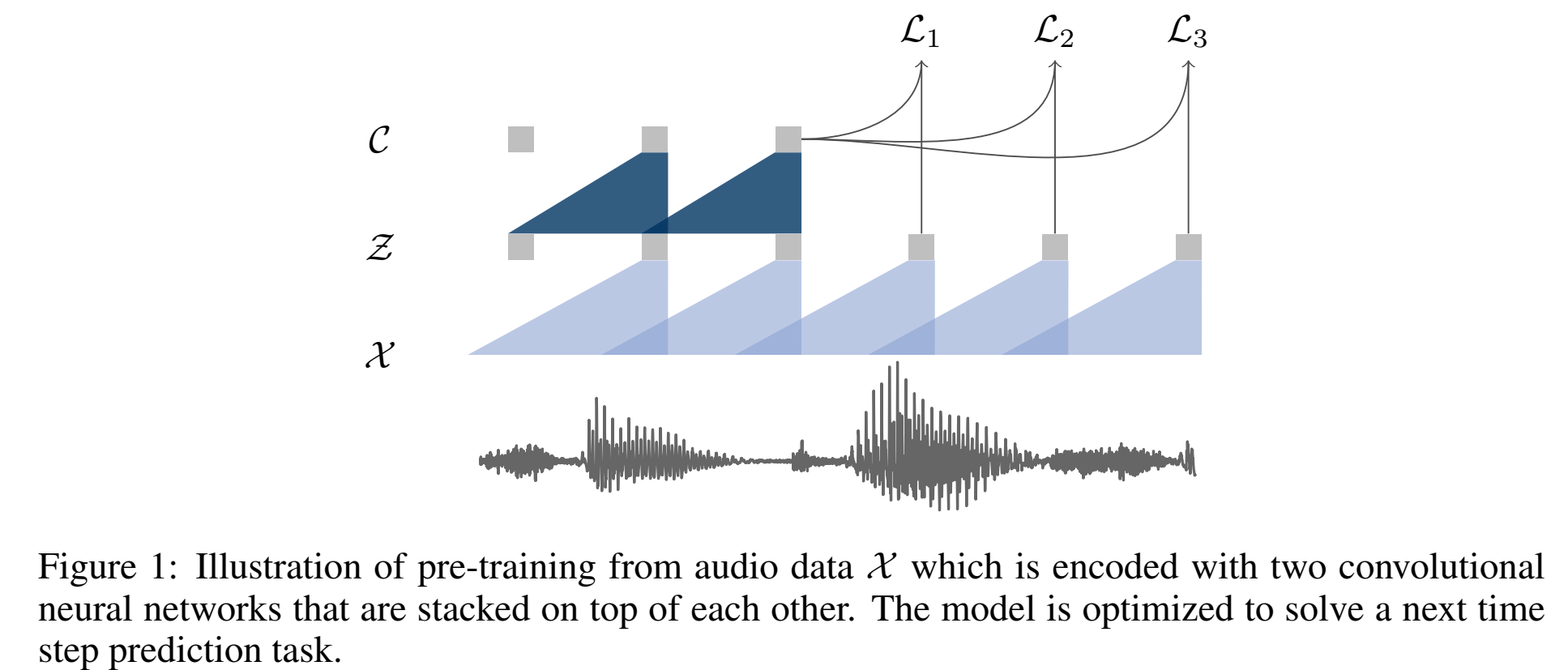
loss function:we optimize our model (§2.1) to predict future samples from a given signal context.
不是对 $p(x)$ 建模,而是在时间轴建模 $\large p(z_{i+k}|z_i…z_{i-r})/p(z_{i+k})$
loss function:
作者利用了负采样技术,作者从一个概率分布 $p_n$ 中采样出负样本 $\tilde z$,,最终模型的loss为区分正例和反例的contrastive loss:
实验
==Baevski, Alexei, et al. “wav2vec 2.0: A framework for self-supervised learning of speech representations.” Advances in Neural Information Processing Systems 33 (2020): 12449-12460.==
==Baevski, Alexei, et al. “Data2vec: A general framework for self-supervised learning in speech, vision and language.” arXiv preprint arXiv:2202.03555 (2022).==
github:https://github.com/pytorch/fairseq/tree/main/examples/data2vec
==Chen, Sanyuan, et al. “Wavlm: Large-scale self-supervised pre-training for full stack speech processing.” arXiv preprint arXiv:2110.13900 (2021).==
中文预训练模型
https://github.com/wenet-e2e/wenet/blob/main/docs/pretrained_models.md
https://github.com/wenet-e2e/wenet/tree/main/examples/openasr2021/s0